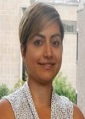
Naz Orang
University of Toronto, Canada
Title: Predictive statistical model for optimized biomass boiler operation
Biography
Biography: Naz Orang
Abstract
Biomass boilers provide pulp mills with one third of their required energy in the form of superheated steam. The biomass fuel in these types of boilers is conventionally called hog fuel, which is a mixture of different types of wood waste found on the mill site. Biomass is considered a carbon neutral fuel and is therefore sustainable and provides a feasible way for mills to dispose of their wood waste while harvesting its energy content. Burning wood waste in biomass boilers is an economical and environmentally sustainable way for pulp mills to remain competitive in today’s challenging market. The variability of hog fuel makes stable and optimized boiler operation a challenge. Since the quality of the fuel is constantly changing, there is a need to detect sub optimal operation and to mitigate the adverse effects of fuel variability as early as possible. For this reason, a predictive statistical model is presented which enables the detection of process upsets caused by changes in hog fuel quality before these changes affect steam production. An OPLS (orthogonal projection to latent structures) model was built for operating data from a stoker-grate biomass boiler in a Canadian pulp mill. Biomass boiler operating data is highly
interconnected and correlated, and OPLS is a predictive projection method which is well suited in dealing with these issues. The thermal performance of the boiler, which is the amount of steam produced per amount of biomass burnt, is modeled as the target variable using operating parameters such as inlet air flow rate and temperature, flue gas flow rate, temperature and composition as predictor variables. The model enables the prediction of the onset of a process upset, which will lead to a decrease in thermal performance and the contributing factors to the upset are also identified by the model which can be used to mitigate the situation and increase thermal performance back to optimal values. This way, biomass combustion for the purpose of steam production can be carried out with minimal co-combustion of fossil fuels.